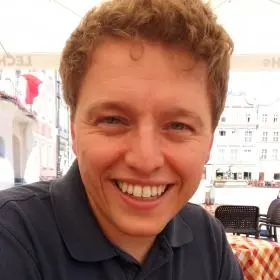
Fill This Form To Receive Instant Help
Words: 1468
Published: May 29, 2024
With an average of 256 programming languages, data scientists have a wide variety of languages to use in their analytical tasks. Some programming languages work best for software application development, other work best for development of games while others work best for data science. Statistical programming languages are high-level programming languages that enable data scientist to create code that is independent of the type of computer. Statistical programming languages are used to analyze data sets and help analysts to generate useful information from big data. Some of the most familiar statistical programming languages include R programming language, SQL, BASH, MATLAB, PHP, Ruby, Go, SAS, Java and Python (Tippmann, 2015). First, statistical programming languages and tools provide data analysts with an effective platform representing, modelling and processing massive amounts of data. The exponential growth in the internet, information systems, communication networks and social media platforms have enabled data scientists to collect massive amounts of structured, semi- structured and unstructured data. Statistical programming languages provide tools and means to derive knowledge, insights and conclusions from the data that may not be in the data set directly.
Secondly, statistical programming languages such as R and Python provide algorithms that help computer scientists to process raw data. These languages offer several analytical methods that reveal interesting patterns and trends from big data. Data scientists use numerous algorithms for machine learning, Artificial Intelligence and predictive analysis. Some of the algorithms include K Nearest Neighbors, K means clustering, Linear Regression and Logistic Regression. Statistical programming languages are also significantly crucial for statistical modelling models. Experts define statistical models as mathematical models that embodies statistical assumptions concerning the generation of sample data, or similar data gathered from larger populations. Statistical modelling helps data scientists to encode information extracted from the data more effectively and quickly.
The accuracy of the conclusions drawn from big data analysis is of paramount importance in the digital world. Statistical programming languages provide a standard form of processing data, thus allowing data scientists from across the globe to collaborate in data processing tasks. The standardization of data analysis also enables the comparison of the results obtained and to determine the accuracy of the knowledge generated (Tippmann, 2015). Despite the complexity of some programming languages such as R, they are relatively easy to use once the analysts understand the syntax of the language. It is relatively easy to generate mine insights from data when using statistical programming languages. Lastly, statistical programming languages allow swift and quick mining of big data. Several programming languages enhance the development of communities that consist of leading statisticians, data analysts and data scientists from different parts of the globe.
R is one of the most popular statistical programming language for statistical modelling and analysis. Like any other programming language, R has its advantages and disadvantages.
References
Keep in mind: This sample was shared by another student.